* This post is in Portuguese.
Há poucos dias, a prefeitura de Porto Alegre liberou os datasets com os dados de despesas de custeio de vários órgãos municipais (Secretaria Municipal de Saúde, Secretaria Municipal de Cultura, Gabinete do Prefeito, etc.). O plot abaixo mostra a quantidade de empenhos para cada órgão municipal:
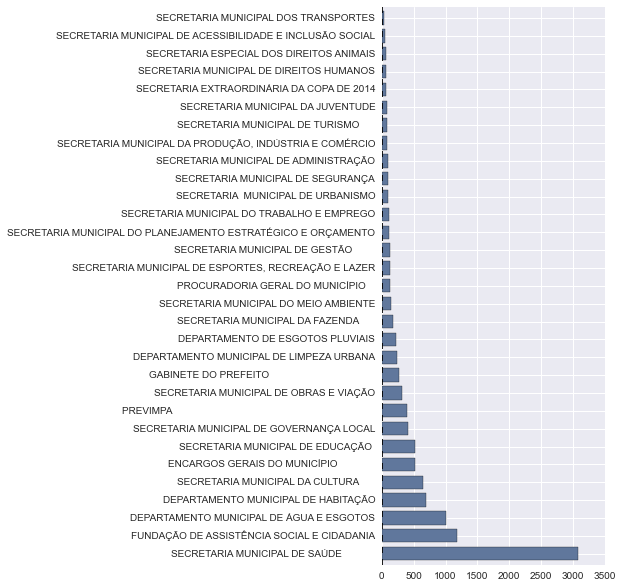
Uma das maneiras utilizadas geralmente para verificar fraudes é o uso da Lei de Benford [1] [2] [3], que fala sobre a distribuição das frequências de dígitos em vários datasets da vida real, incluindo valores de ações, número de populações, tamanhos de rios, etc.
Ao correlacionar a distribuição de números dos primeiros digitos dos valores de empenhos dos dados de Despesas de Custeio do 2º bimestre de 2014 com a distribuição da Lei de Benford, a correlação ficou muito clara:
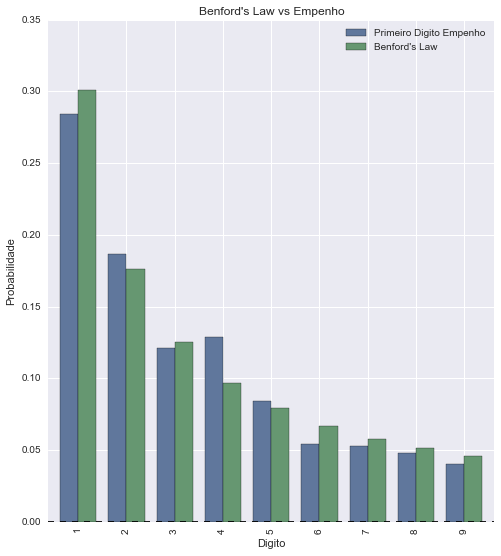
Segue aí mais um exemplo de correlação da Lei de Benford. Um sistema legal para ser construído seria um monitor de despesas que verificasse a correlação da Lei de Benford automaticamente e alertasse a cada anomalia encontrada.