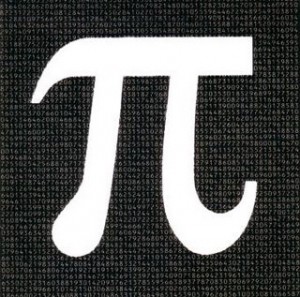
As many (or very few in the real life haha) people know, today is the Pi Approximation Day ! So it’s time to make a contribution to celebrate this funny day =)
My contribution is to use Python and Pyevolve to approximate Pi number using Genetic Programming approach. I’ve created the functions gp_add(+), gp_sub(-), gp_div(/), gp_mul(*) and gp_sqrt (square root) to use as non-terminals of the GP. The fitness function is very simple too, it simple returns the absolute difference between the Python math.pi and the evaluated individual. I’ve used also a population size of 1k individuals with max tree depth of 8 and the random ephemeral constants as random integers. The best approximation I’ve got while running the GP for about 8 minutes (40 generations) was 3.1416185511, best for 3 digits, you can improve it and let it run for more time to get better approximations.
Here is the formulae I’ve got with the GP (click to enlarge):
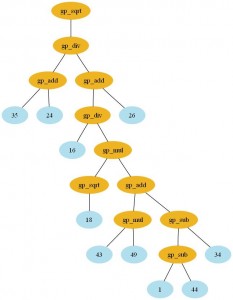
And here is the output of the script:
Best (0): 3.1577998365
Error: 0.0162071829
Best (10): 3.1417973679
Error: 0.0002047143
Best (20): 3.1417973679
Error: 0.0002047143
Best (30): 3.1417973679
Error: 0.0002047143
Best (40): 3.1416185511
Error: 0.0000258975
- GenomeBase
Score: 0.000026
Fitness: 15751.020831
Params: {'max_depth': 8, 'method': 'ramped'}
Slot [Evaluator] (Count: 1)
Slot [Initializator] (Count: 1)
Name: GTreeGPInitializator - Weight: 0.50
Doc: This initializator accepts the follow parameters:
*max_depth*
The max depth of the tree
*method*
The method, accepts "grow" or "full"
.. versionadded:: 0.6
The *GTreeGPInitializator* function.
Slot [Mutator] (Count: 1)
Name: GTreeGPMutatorSubtree - Weight: 0.50
Doc: The mutator of GTreeGP, Subtree Mutator
.. versionadded:: 0.6
The *GTreeGPMutatorSubtree* function
Slot [Crossover] (Count: 1)
Name: GTreeGPCrossoverSinglePoint - Weight: 0.50
- GTree
Height: 8
Nodes: 21
GTreeNodeBase [Childs=1] - [gp_sqrt]
GTreeNodeBase [Childs=2] - [gp_div]
GTreeNodeBase [Childs=2] - [gp_add]
GTreeNodeBase [Childs=0] - [26]
GTreeNodeBase [Childs=2] - [gp_div]
GTreeNodeBase [Childs=2] - [gp_mul]
GTreeNodeBase [Childs=2] - [gp_add]
GTreeNodeBase [Childs=2] - [gp_sub]
GTreeNodeBase [Childs=0] - [34]
GTreeNodeBase [Childs=2] - [gp_sub]
GTreeNodeBase [Childs=0] - [44]
GTreeNodeBase [Childs=0] - [1]
GTreeNodeBase [Childs=2] - [gp_mul]
GTreeNodeBase [Childs=0] - [49]
GTreeNodeBase [Childs=0] - [43]
GTreeNodeBase [Childs=1] - [gp_sqrt]
GTreeNodeBase [Childs=0] - [18]
GTreeNodeBase [Childs=0] - [16]
GTreeNodeBase [Childs=2] - [gp_add]
GTreeNodeBase [Childs=0] - [24]
GTreeNodeBase [Childs=0] - [35]
- GTreeGP
Expression: gp_sqrt(gp_div(gp_add(26,
gp_div(gp_mul(gp_add(gp_sub(34,
gp_sub(44, 1)), gp_mul(49, 43)), gp_sqrt(18)),
16)), gp_add(24, 35)))
And finally, here is the source code:
from __future__ import division
from pyevolve import *
import math
def gp_add(a, b): return a+b
def gp_sub(a, b): return a-b
def gp_div(a, b): return 1 if b==0 else a/b
def gp_mul(a, b): return a*b
def gp_sqrt(a): return math.sqrt(abs(a))
def eval_func(chromosome):
code_comp = chromosome.getCompiledCode()
ret = eval(code_comp)
return abs(math.pi - ret)
def step_callback(engine):
gen = engine.getCurrentGeneration()
if gen % 10 == 0:
best = engine.bestIndividual()
best_pi = eval(best.getCompiledCode())
print "Best (%d): %.10f" % (gen, best_pi)
print "\tError: %.10f" % (abs(math.pi - best_pi))
return False
def main_run():
genome = GTree.GTreeGP()
genome.setParams(max_depth=8, method="ramped")
genome.evaluator += eval_func
ga = GSimpleGA.GSimpleGA(genome)
ga.setParams(gp_terminals = ['ephemeral:random.randint(1, 50)'],
gp_function_prefix = "gp")
ga.setMinimax(Consts.minimaxType["minimize"])
ga.setGenerations(50000)
ga.setCrossoverRate(1.0)
ga.setMutationRate(0.09)
ga.setPopulationSize(1000)
ga.stepCallback.set(step_callback)
ga.evolve()
best = ga.bestIndividual()
best.writeDotImage("tree_pi.png")
print best
if __name__ == "__main__":
main_run()
If you are interested why today is the Pi Approximation day, see some resources:
Little Cartoon
Some Background History
Some Pi Approximations